Unlocking the Hidden Costs: The True Cost of Bad Data and How It Can Hinder the Benefits of AI
- AlyData
- Sep 5, 2023
- 6 min read
Introduction: The Ripple Effect of Bad Data on AI
The increasing dependence on Artificial Intelligence (AI) is undeniable. From businesses to healthcare, AI has emerged as a groundbreaking technology that promises efficiency, effectiveness, and transformative solutions. However, just like any sophisticated machine, AI is only as good as the data fed into it. Poor-quality or "bad" data can have a domino effect that magnifies the limitations of AI, leading to faulty outcomes and substantial costs for businesses. This article will unpack the true cost of bad data and explore how it can hinder the benefits of AI.
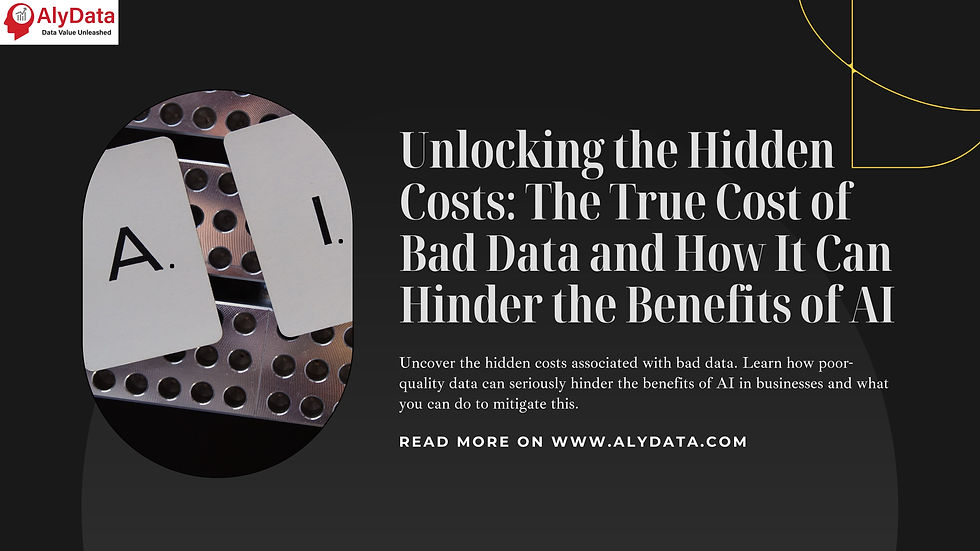
What is Bad Data?
Bad data, in simple terms, is information that is incorrect, incomplete, outdated, or irrelevant. Imagine constructing a building on a shaky foundation; bad data serves as that faulty base. You may have all the architectural prowess in the world, but your construction is bound to collapse if the foundational elements are flawed. When this bad data becomes the basis for machine learning algorithms or AI systems, the results can be disastrous.
Types of Bad Data
Understanding the different kinds of bad data can help in diagnosing and fixing the problem at its root. Some of the common types are:
Duplicate Data: This occurs when the same data entry appears more than once.
Inaccurate Data: Information that is wrong or misleading.
Outdated Data: Old information that has not been updated.
Inconsistent Data: When data is recorded in various formats or units, causing discrepancies.
By recognizing these types, businesses can take the first steps toward ensuring that their data is clean, reliable, and usable for AI applications.
The Genesis of Bad Data
So, where does bad data come from? While there’s no single answer, bad data often originates from human error, system glitches, or a lack of standard operating procedures for data entry and maintenance. Additionally, the rapid influx of data from various sources—social media, sensors, customer feedback—can overwhelm systems, leading to the storage of bad data.
Is All Data Useful?
Contrary to popular belief, not all data is useful. The indiscriminate hoarding of data can clog systems and make it difficult to distinguish between valuable information and useless noise. Imagine searching for a needle in a haystack; the excess of irrelevant data only makes the task more arduous.
The Costs of Bad Data
The repercussions of bad data can be severe. According to Gartner, poor-quality data can cost businesses an average of $9.7 million per year. The financial aspect is just the tip of the iceberg; the hidden costs of reputational damage, lost opportunities, and regulatory fines can be even more crippling.
Why Businesses Struggle with Bad Data
Data management is no small feat. Many businesses, especially small to medium enterprises, often lack the expertise, technology, and resources to maintain high-quality data. Ignorance or underestimation of the implications of bad data can also contribute to this problem.
The Role of Bad Data in Poor Decision-making
Decisions rooted in bad data can have devastating outcomes for a business. Incorrect insights can lead to poor strategy, which can cascade into a cycle of failures and losses. Moreover, bad data can send the company down a rabbit hole of inaccurate forecasting, eventually derailing growth.
Bad Data and Wasted Resources
Time and resources spent on correcting or cleaning bad data are significant. The manpower involved in manually rectifying mistakes, the time taken to reconcile inconsistencies, and the opportunity cost of missed chances all contribute to the toll that bad data takes on a company.
How Bad Data Affects Customer Experience
Imagine sending promotional emails to the wrong audience or recommending irrelevant products to a customer. Such blunders, rooted in bad data, can lead to customer dissatisfaction, lost sales, and tarnished brand image.
The True Cost of Bad Data and How It Can Hinder the Benefits of AI
AI has the potential to revolutionize various aspects of business, from customer relations to supply chain management. However, bad data serves as kryptonite to these superpowers. An AI system trained on flawed data will only produce flawed results, squandering the immense benefits this technology can offer.
AI’s Role in Amplifying the Costs of Bad Data
AI systems are particularly sensitive to the quality of data. Incorrect data not only leads to wrong predictions but also corrupts the AI model, rendering it useless or even harmful. The very tool that is meant to streamline operations and provide insightful analyses can turn into a liability if contaminated with bad data.
Case Studies: When Bad Data Meets AI
Several cases demonstrate the catastrophic outcomes when AI is fed with bad data. For instance, a healthcare AI system that wrongly predicted patient risks led to incorrect treatments and endangered lives. Another case involved a financial AI tool that advised on risky investments, causing significant losses for the company.
Financial Losses Due to Bad Data
As mentioned earlier, the financial impact can be debilitating. From the costs incurred in data cleansing to lost revenues from poor decision-making, the monetary consequences are severe.
Regulatory Risks and Legal Consequences
Bad data can also expose a company to legal risks. Incorrect reporting or breach of data integrity requirements can result in hefty fines and legal action.
How to Identify Bad Data
Identifying bad data is the first step toward resolution. Regular data audits, cross-referencing sources, and setting quality benchmarks can aid in this process.
Data Auditing Techniques
A thorough data audit involves checking for inaccuracies, inconsistencies, and irrelevant information. Various software tools can help automate this process, making it more efficient and reliable.
The Importance of Data Quality Management
Quality management isn't just for manufacturing or customer service; it's crucial for data as well. A comprehensive data quality management strategy can help your business ensure that the data you collect and use is accurate, consistent, and actionable. The absence of such a strategy can lead to the accumulation of bad data, which we've established can lead to a wide array of issues, from skewed analytics to poor customer experiences.
Data Governance Policies
Data governance isn't just a buzzword; it's a necessity. Establishing a set of rules and processes for how data is collected, stored, and accessed can go a long way in preventing the introduction of bad data into your systems. Whether it's setting up data validation checks or defining who has the authorization to access and modify data, governance policies serve as the guardrails that keep your data quality on track.
Technologies that Can Improve Data Quality
Luckily, businesses today have a variety of technologies at their disposal to tackle the issue of bad data. Data quality software, data lakes, and even certain AI-powered tools can streamline the process of cleaning and maintaining high-quality data. For example, machine learning algorithms can automatically detect and correct anomalies in data sets, saving time and reducing human error.
Tips for Maintaining High-Quality Data
Maintaining high-quality data is a continuous process. A few best practices include regular audits, training staff on the importance of data quality, and keeping abreast of the latest technologies and methods for data quality improvement. Above all, a proactive approach to data quality is always better than a reactive one.
The Future of Data Quality and AI
As AI technologies evolve, so do the tools for managing data quality. Future trends indicate a more integrated approach, where AI systems themselves will have built-in data quality checks. This symbiosis between AI and data quality will be a game-changer in the efficient management and application of business data.
How Businesses Can Leverage AI for Better Data Quality
AI isn't just a victim of bad data; it can be part of the solution. Machine learning algorithms can be trained to identify bad data, automate the cleaning process, and even predict where bad data is likely to occur. Businesses can leverage this capability to not just solve existing data quality issues, but also preempt future ones.
Future Technologies to Watch For
Blockchain, edge computing, and augmented analytics are just a few of the technologies that promise to revolutionize the way we think about data quality. These technologies offer more secure, faster, and smarter ways to manage data, which will, in turn, enhance the quality of the data that feeds into AI algorithms.
Conclusion: The Path Forward
Bad data is not just an IT issue; it's a business problem with far-reaching consequences. Understanding the true cost of bad data and its impact on the benefits of AI is the first step toward a sustainable solution. The key lies in treating data as a valuable asset, investing in robust data governance and quality management strategies, and adopting cutting-edge technologies that can help maintain the quality of this asset.
Frequently Asked Questions
What is bad data? Bad data refers to information that is inaccurate, incomplete, outdated, or irrelevant. It can severely affect the performance of AI systems and lead to poor business decisions.
Why is bad data a problem for AI? AI systems rely heavily on data for learning and making predictions or decisions. Poor-quality data can corrupt these processes, leading to inaccurate or even harmful outcomes.
How does bad data affect businesses? The impacts range from financial losses to operational inefficiencies, poor customer experiences, and even legal consequences.
What steps can businesses take to improve data quality? Regular data audits, employee training, and the use of advanced technologies can all contribute to better data quality.
Can AI help in improving data quality? Yes, AI technologies can be leveraged to automate the data cleaning process and to identify potential areas where bad data could occur.
What is data governance? Data governance involves setting up rules and procedures for collecting, storing, and accessing data, helping maintain its quality over time.
Comments