Underlining the Importance of Data Governance Through the Lens of AI Model Collapse
- AlyData
- Jul 18, 2023
- 3 min read
Artificial intelligence (AI) has become an integral part of our daily lives, transforming various aspects of society. However, with its wide adoption comes the risk of AI model collapse, where these intelligent systems start generating erroneous or biased outcomes. In this blog post, we will explore the significance of data governance in mitigating the risks associated with AI model collapse.
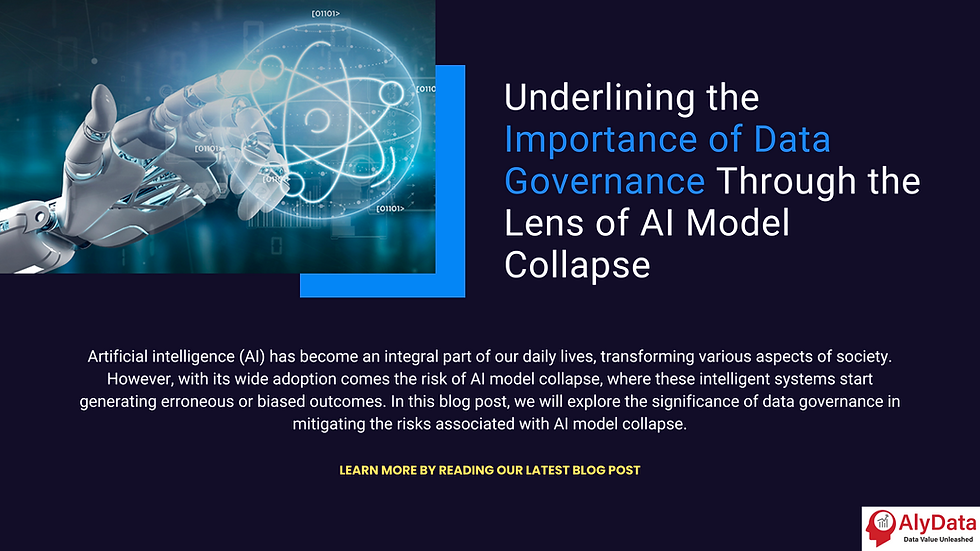
Understanding AI Model Collapse
AI model collapse refers to a situation where an AI model starts producing increasingly inaccurate or biased results. Several factors can trigger such collapses, including the use of low-quality data, data with inherent bias, or the adoption of an unsuitable model for the task at hand. The consequences of an AI model collapse can be severe, particularly for businesses and organizations heavily reliant on AI.
The Implications of AI Model Collapse
When an AI model collapses, it can have far-reaching implications. For example, in a financial decision-making AI model, a collapse could lead to substantial financial losses. Similarly, in an AI model used for job applicant screening, a collapse could inadvertently perpetuate discrimination against certain demographic groups. Therefore, it is crucial for organizations to take proactive measures to prevent and mitigate the risk of AI model collapse.
The Role of Data Governance in Preventing AI Model Collapse
To minimize the risk of AI model collapse, organizations need to implement robust data governance strategies. Data governance involves managing the flow of data within an organization, encompassing aspects such as data collection, storage, analysis, and security. By adhering to effective data governance practices, organizations can ensure that AI models are trained on unbiased, high-quality data, reducing the likelihood of collapse.
Here's a look at how data governance can specifically assist in reducing the risk of AI model collapse:
Data Collection: Implementing a consistent and structured data collection process through data governance enables easy AI model training and early detection of data-related issues.
Data Storage: Secure storage practices and controlled data access, integral parts of data governance, safeguard data from unauthorized access or alteration, thus preventing potential AI model collapse.
Data Analysis: Data governance helps align data analysis with the organization's objectives, ensuring AI models are trained on task-relevant data.
Data Security: By implementing data security measures through data governance, organizations protect data from unauthorized access, reducing the chances of biased or inaccurate AI models.
Strategies for Incorporating Data Governance in AI
To effectively incorporate data governance in AI initiatives, organizations should consider the following strategies:
Recognize your data assets: Identify all data assets within your organization, including structured and unstructured data, to understand the scope and quality of available data.
Evaluate your data quality: Assess the quality of your data by detecting any errors or inconsistencies, ensuring that AI models are trained on reliable and accurate information.
Establish a data governance framework: Develop a comprehensive data governance framework that outlines the norms and procedures for managing and safeguarding your organization's data.
Supervise your data governance program: Continuously monitor the effectiveness of your data governance program, track adherence to the established framework, and identify areas that require improvement.
By adhering to these strategies, organizations can manage their data in a manner that reduces the risk of AI model collapse, promoting the safe and responsible use of AI technologies.
In conclusion, data governance plays a critical role in mitigating the risks associated with AI model collapse. By implementing robust data governance strategies, organizations can ensure that AI models are trained on unbiased and high-quality data, minimizing the potential for erroneous or biased outcomes. It is imperative for businesses and organizations to prioritize data governance to foster the responsible and effective use of AI.
Comments