Explore how inadequate data governance hinders AI integration in enterprises. Understand the significance, impacts, and solutions for effective AI strategy.
In the modern enterprise world, Artificial Intelligence (AI) has taken center stage, offering immense potential for businesses in various sectors. However, a significant roadblock that impedes the widespread adoption of AI in enterprises is the lack of data governance. In this comprehensive guide, we will delve deep into why proper data governance is paramount and how its absence can hinder enterprise AI adoption.
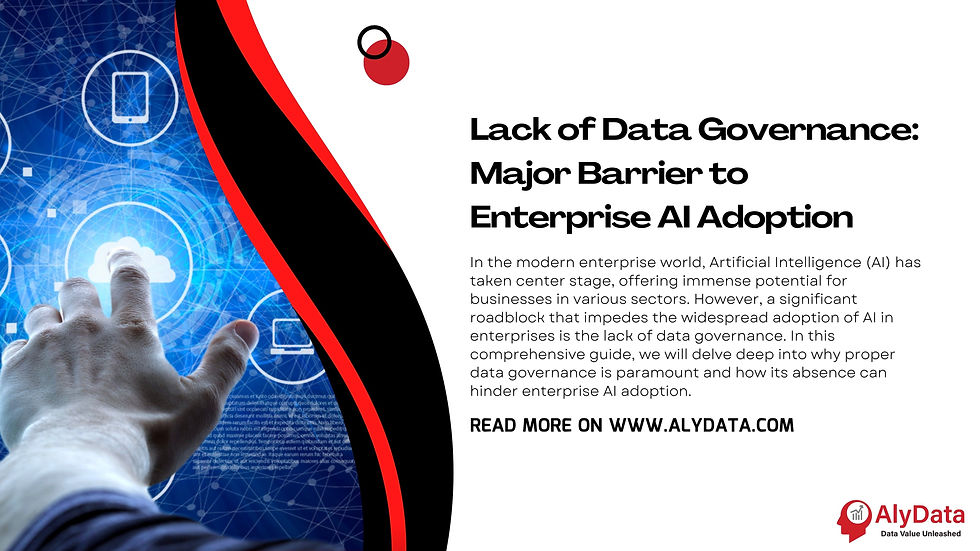
Understanding the Significance of Data Governance
At the core of any AI solution lies data – the richer and more accurate this data, the better the AI's performance. Data governance is the holistic management of data availability, usability, integrity, and security. Without proper data governance in place, businesses risk feeding their AI systems with flawed, inconsistent, or biased data, leading to inaccurate or unreliable outputs.
The Direct Impact of Poor Data Governance on AI
Inconsistent Data Quality: Without standardization, data can become fragmented and inconsistent. This inconsistency can severely compromise the AI model's learning process, leading to inaccurate predictions or recommendations.
Reduced Trust in AI Systems: When AI outputs are based on poorly governed data, the end results can be questionable. This can lead to stakeholders losing trust in AI-driven decisions, rendering the entire AI investment moot.
Increased Costs: Constantly rectifying errors resulting from poorly governed data can lead to higher operational costs. Moreover, if the AI system makes a significant error due to poor data, the costs associated with rectifying such mistakes can be substantial.
Regulatory and Compliance Issues: With global regulations like GDPR in place, mishandling data can lead to hefty fines and legal repercussions. Proper data governance ensures compliance with such regulations, safeguarding businesses from potential legal pitfalls.
Key Components of Effective Data Governance for AI
To facilitate seamless AI adoption in enterprises, there are some pivotal components of data governance that businesses must pay heed to:
Data Quality Management: This involves standardizing, cleaning, and validating data to ensure it's accurate, complete, and timely. A robust data quality management system can significantly enhance the reliability of AI predictions.
Data Access Control: By defining who has access to what data, businesses can ensure data security and privacy. This is crucial, especially when dealing with sensitive information.
Data Lineage and Metadata Management: Understanding where data comes from and how it's processed can aid in troubleshooting and refining AI models. Metadata, the data about data, helps in understanding its context and relevance.
Data Lifecycle Management: This includes the policies and processes associated with data creation, storage, usage, and deletion. Ensuring a systematic data lifecycle management can aid in maintaining data's relevance and freshness for AI models.
The Path Forward: Integrating Data Governance with AI Strategy
For businesses aspiring to leverage the full potential of AI, integrating data governance into their AI strategy is non-negotiable. Here are some steps enterprises can take:
Establish a Dedicated Data Governance Team: Comprising data scientists, IT professionals, and domain experts, this team can ensure that data governance policies are formulated and adhered to.
Invest in Data Governance Tools: Leveraging advanced tools can automate many aspects of data governance, ensuring consistency and efficiency.
Regular Audits and Reviews: Periodically reviewing the data governance policies can help in identifying gaps and refining them for better AI performance.
Employee Training: Making employees aware of the importance of data governance and training them in best practices can foster a culture of data-centricity in the organization.
In the realm of enterprise AI, the adage "garbage in, garbage out" holds exceptionally true. The success of AI in any organization hinges on the quality and integrity of data fed into it. Thus, prioritizing data governance is not just a best practice; it's a necessity for businesses aiming for successful AI adoption.
Comments