Empowering Organizations: Creating Proprietary Large Language Models with Enterprise Data
- AlyData
- Jul 12, 2023
- 3 min read
The field of natural language processing has been greatly impacted by the advent of large language models, which have facilitated the exploitation of text generation and analysis by enterprises. Although there exist pre-trained models such as GPT-3 that have displayed remarkable capabilities, organizations can gain an edge by developing their own large language models by using their exclusive data. This article delves into how organizations can initiate the process of building their own large language models based on their unique data resources, which in turn can unlock novel opportunities, augment their decision-making ability, and help them become more competitive.
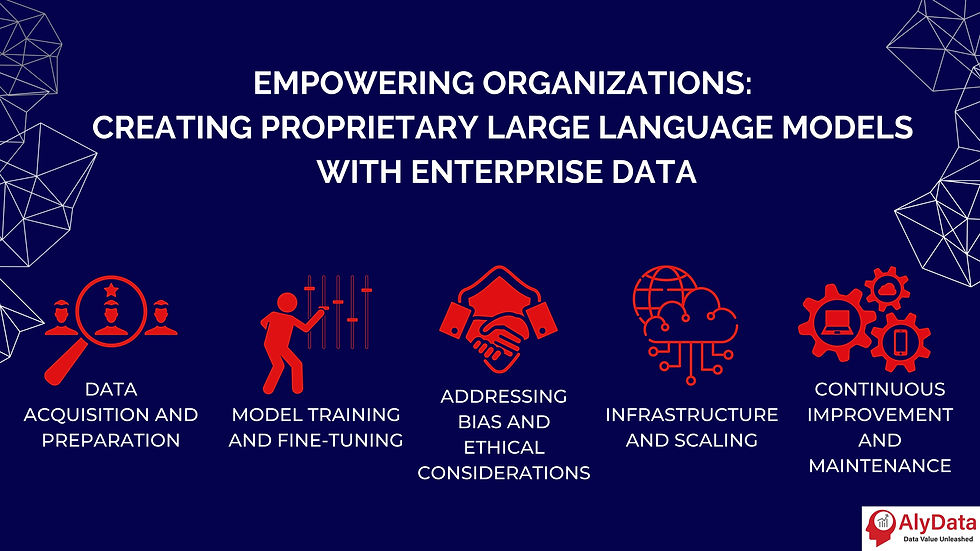
Data Acquisition and Preparation: To embark on the journey of creating a proprietary large language model, the initial step is to source and organize the data. Enterprises can capitalize on their existing data repositories such as customer interactions, support tickets, internal communications, and documents. This data must undergo meticulous curation, guaranteeing its pertinence, precision, and caliber. It may entail a process of data cleaning, anonymization, and framework to erase personally identifiable information and assure compliance with data privacy regulations.
Model Training and Fine-tuning: Post curating the data, corporations can then move on to the model training and fine-tuning phase. This includes picking out an appropriate machine learning framework - TensorFlow or PyTorch, for instance, and deploying sophisticated mechanisms like deep learning and transformer architectures. The data is used to train the model, enabling it to decipher patterns, comprehend context, and generate text in accordance with the organization's specific domain or use case. Enhancing the model's efficacy necessitates several iterations and training cycles to optimize its overall performance.
Addressing Bias and Ethical Considerations: During the course of model development, organizations must actively tackle bias and ethical apprehensions. This encompasses assessing and diminishing biases present in the training data, ensuring impartiality, and examining the ethical implications of the generated content. By utilizing varied and comprehensive datasets, corporations can lower biases and fabricate models with just and unbiased outcomes. Ongoing surveillance and assessment are indispensable to detect and rectify biases that may crop up during training and fine-tuning.
Infrastructure and Scaling: Effectuating large language models demands sturdy infrastructure and scaling abilities. In order to ensure successful model training and deployment, organizations must assess the computational resources, storage, and infrastructure necessary for the process. Cloud computing platforms such as AWS, Google Cloud, or Azure provide adjustable solutions to accommodate the computational demands of developing large language models on a large scale. Organizations can work in tandem with data scientists, machine learning engineers, and cloud service providers to fine-tune their infrastructure and guarantee expeditious model training and deployment.
Continuous Improvement and Maintenance: Developing proprietary large language models is a perpetual undertaking that necessitates unceasing refinement and upkeep. Organizations should institute a feedback loop to acquire valuable insights and feedback from users, enabling them to iteratively improve and augment the models over time. Regular updates, fine-tuning, and retraining are imperative for accommodating dynamic data patterns, industry trends, and user demands. Furthermore, organizations must remain up-to-date with the latest research and advancements in natural language processing to ensure the models stay at the forefront of technological progress.
Developing proprietary large language models empowers organizations to harness their distinctive data assets and bolster their proficiency in natural language processing. By acquiring and curating pertinent data, undertaking model training and fine-tuning, addressing biases, prioritizing ethical considerations, optimizing infrastructure, and emphasizing continuous improvement, organizations can mold models tailored to their precise requirements and domains. These exclusive large language models grant organizations the capacity to produce contextually precise and personalized insights, ultimately fostering better decision-making, enriched customer experiences, and a distinct competitive advantage in the contemporary data-driven landscape.
About AlyData
Our company's mission is to revolutionize organizations by facilitating innovation and providing a competitive edge through the realization of tangible business value from their data and information assets.
AlyData (http://www.alydata.com) specializes in CDO Advisory, Data Management (i.e., Data & AI Governance, Data Quality, Data Catalog, Master Data Management, Data Privacy and Security, and Metadata Management), and Data Science/Artificial Intelligence. If your organization is grappling with data silos, struggling with data complexity, and requires a reliable partner to drive business outcomes, please get in touch with us via https://calendly.com/jayzaidi-alydata.
AlyData has a strategic partnership with Collibra and has demonstrated expertise in developing the strategy, roadmap, and implementation of Collibra's Data Governance, Data Privacy, and Data Quality modules for clients.
Comments