7 Crucial Aspects of Data Quality for Stellar AI Outcomes
- AlyData
- Aug 22, 2023
- 3 min read
Introduction
In the transformative age of Artificial Intelligence (AI), we're often caught up in algorithms and fancy buzzwords. However, the heart of effective AI lies somewhere far more foundational: data quality. In this guide, we’ll unpack the immense importance of data quality for superior AI outcomes, highlighting why it's the unsung hero of any successful AI initiative.
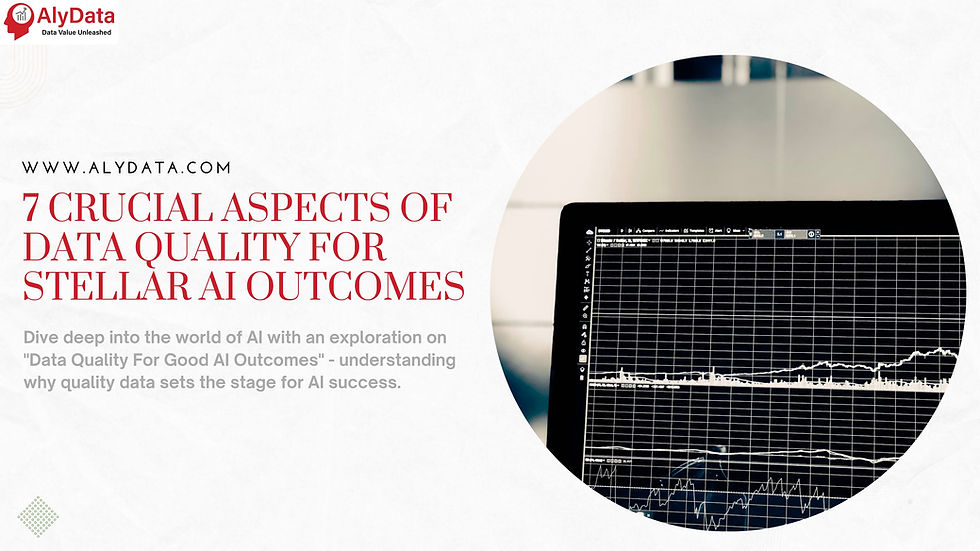
Data Quality For Good AI Outcomes: Why It's the Gold Standard
Data, often referred to as the "new oil", serves as the backbone for AI systems. The adage, "garbage in, garbage out", perfectly encapsulates the essence of why data quality is paramount. Without pristine data, even the most sophisticated AI models falter, rendering outcomes that are at best unreliable and at worst detrimental.
Dimensions of Data Quality
When discussing data quality, it’s not just about clean data, but rather a multi-faceted spectrum of characteristics:
Accuracy: Does your data reflect reality?
Completeness: Are any data points missing?
Consistency: Are datasets uniform across the board?
Timeliness: Is your data up-to-date?
Uniqueness: Are there unnecessary duplications?
Each dimension plays a pivotal role in ensuring that AI models churn out outcomes that are robust, reliable, and reflective of the real world.
Impact of Poor Data Quality
Imagine a self-driving car trained on inaccurate data. The consequences could be catastrophic. Poor data quality not only tarnishes the reliability of AI systems but can pose genuine risks, especially in critical sectors like healthcare, finance, and transportation.
The Interplay of Volume and Quality
It's a myth that more data guarantees better AI outcomes. While volume can provide a richer substrate, without the cornerstone of quality, this vastness is futile. In fact, a smaller, high-quality dataset might yield better results than a vast, messy one.
Achieving Data Purity: Best Practices
Curating high-quality data isn’t a stroke of luck but a result of meticulous processes:
Data Cleaning: Remove inaccuracies and inconsistencies.
Data Enrichment: Augment datasets with external sources.
Data Validation: Confirm data's accuracy post-cleaning.
Data Updation: Ensure data remains current with regular updates.
By embedding these practices, organizations can lay a sturdy foundation for AI models that deliver impeccable outcomes.
The Role of Human Oversight
While AI can assist in data quality control, human oversight remains irreplaceable. Human intuition can catch nuances that might elude algorithms, making it a potent ally in the quest for pristine data.
Future of Data and AI: A Symbiotic Relationship
As AI continues to evolve, its reliance on high-quality data will intensify. It's a symbiotic relationship where AI thrives on quality data, and in return, it offers transformative solutions. This synergy underlines the narrative of the future, reiterating that data quality will remain non-negotiable.
FAQs
Why is data quality essential for AI? Data quality ensures AI systems operate as intended, producing reliable and accurate results. It serves as the foundation upon which AI models are built and trained.
How does poor data quality impact AI outcomes? Poor data quality can lead to unreliable results, potentially causing significant harm, especially in critical sectors. For example, in healthcare, it could lead to misdiagnoses.
What are the dimensions of data quality? Data quality encompasses several dimensions, including accuracy, completeness, consistency, timeliness, and uniqueness.
How can organizations ensure their data is of high quality? Organizations can invest in data cleaning, enrichment, validation, and regular updation processes to maintain high data quality.
Why can't we solely rely on AI for data quality? While AI is powerful, human intuition can catch nuances and intricacies that might be overlooked by algorithms, making human oversight crucial.
Is more data always better for AI?
Not necessarily. The quality of data is more important than sheer volume. A smaller, high-quality dataset can sometimes produce better results than a larger, inferior one.
Conclusion
As we steer deeper into the AI-driven era, understanding and acknowledging the pivotal role of data quality becomes crucial. Organizations looking to harness the full potential of AI must prioritize data quality, ensuring their AI models aren't just running, but sprinting towards excellence. Remember, in the intricate dance of AI, data quality leads while the algorithms follow.
Comentarios